Abstract
We aim to understand how actions are performed and identify subtle differences, such as ‘fold firmly’ vs. ‘fold gently’. To this end, we propose a method which recognizes adverbs across different actions. However, such fine-grained annotations are difficult to obtain and their long-tailed nature makes it challenging to recognize adverbs in rare action-adverb compositions. Our approach therefore uses semi-supervised learning with multiple adverb pseudo-labels to leverage videos with only action labels. Combined with adaptive thresholding of these pseudo-adverbs we are able to make efficient use of the available data while tackling the long-tailed distribution. Additionally, we gather adverb annotations for three existing video retrieval datasets, which allows us to introduce the new tasks of recognizing adverbs in unseen action-adverb compositions and unseen domains. Experiments demonstrate the effectiveness of our method, which outperforms prior work in recognizing adverbs and semi-supervised works adapted for adverb recognition. We also show how adverbs can relate fine-grained actions.
Talk
Qualitative Results
Poster
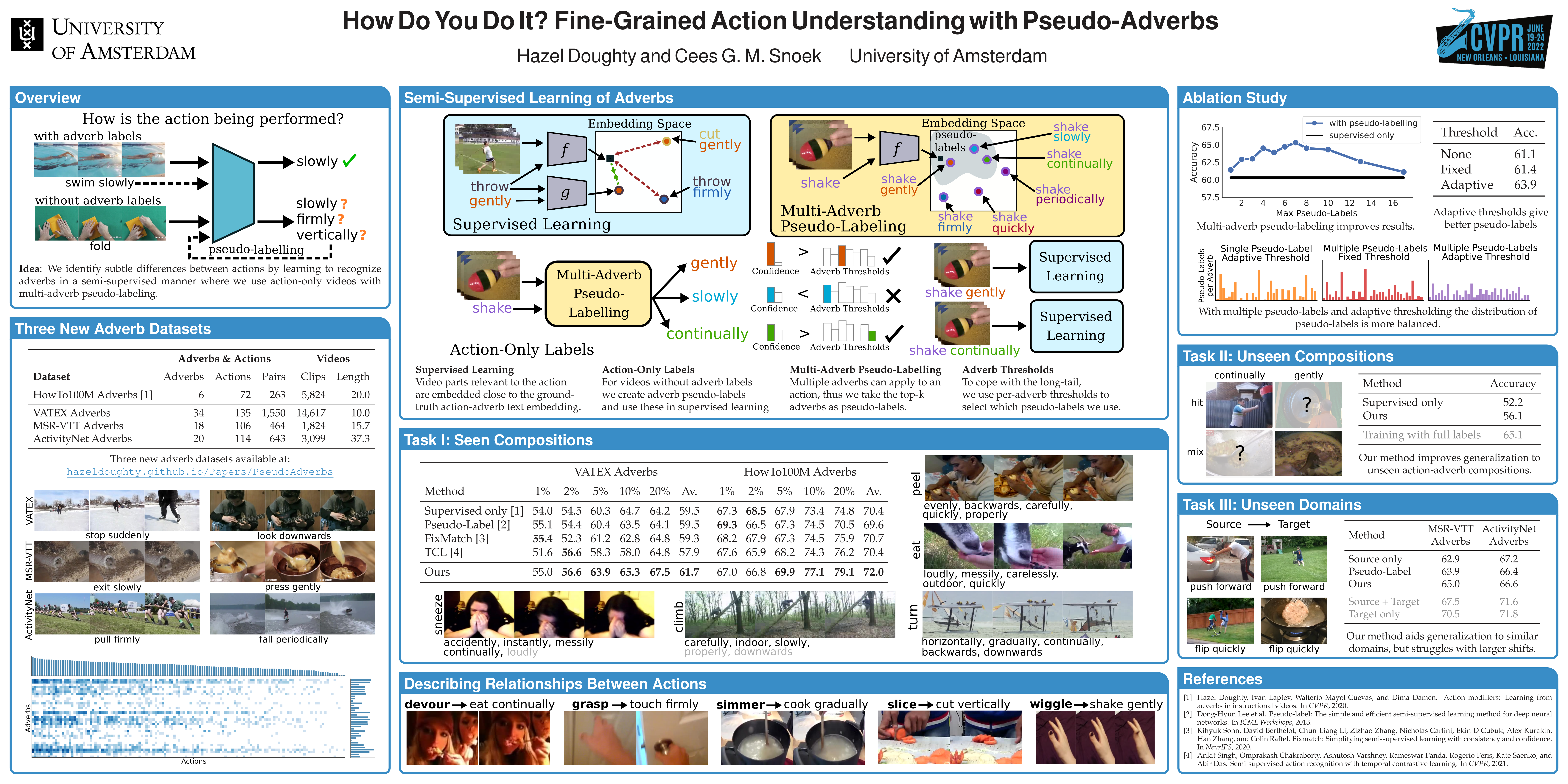
Datasets
In this work we create three new adverb datasets from existing video retrieval datasets: VATEX Adverbs, MSR-VTT Adverbs and ActivityNet Adverbs. These contain less noise and a greater variety of adverbs than existing adverb datasets. VATEX Adverbs is the largest with ~15,000 video clips of 34 adverbs appearing across 135 actions to form 1,550 unique action-adverb pairs. These new datasets allow evaluation of adverb recognition in three settings: action-adverb compositions seen in training, action-adverb compositions which are unseen in training and recognizing adverbs in unseen domains.
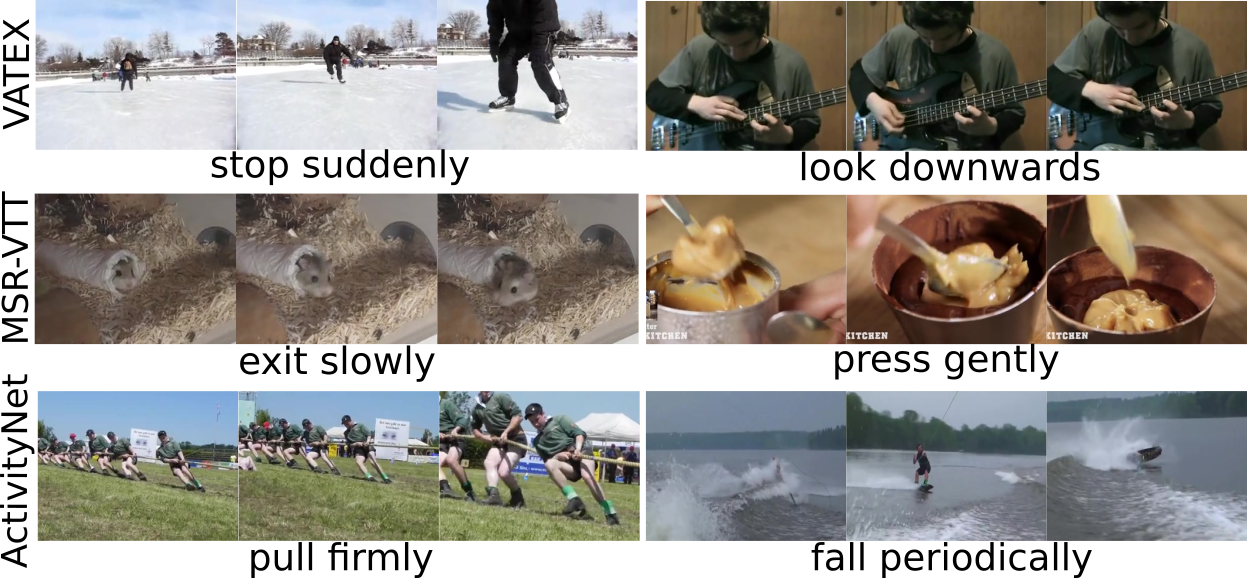
Downloads
Bibtex
@inproceedings{doughty2022how, author = {Doughty, Hazel and Snoek, Cees G. M.}, title = {{H}ow {D}o {Y}ou {D}o {I}t? {F}ine-{G}rained {A}ction {U}nderstanding with {P}seudo-{A}dverbs}, booktitle = {The IEEE/CVF Conference on Computer Vision and Pattern Recognition (CVPR)}, year = {2022} }
Related
Acknowledgements
This work is part of the project Real-Time Video Surveillance Search with project number 18038, which is (partly) financed by the Dutch Research Council (NWO) domain Applied and Engineering/Sciences (TTW).